Problem Statement
It is increasingly tedious for pharmaceutical companies to discover and use internal and external knowledge effectively. They deal with vast amounts of structured and unstructured data from various sources, such as electronic health records, regulatory documents, or clinical trials. Integrating, harmonizing, and governing such heterogeneous and siloed data are significant challenges which need to be overcome whilst complying with regulatory standards and guidelines. Knowledge graphs can be a powerful tool to address those challenges by serving as a unified data model, breaking down silos and contextualizing information with the aim of interconnecting data, improving decision making and ultimately accelerating innovation. This effect can even be amplified when Large Language Models (LLMs) team up with the Knowledge Graph. This article describes how the combination of both can further unleash the potential of Data Governance.
Knowledge Graph (KG)
A knowledge graph is a semantic representation of a particular domain of knowledge from the real world. In contrast to the traditional way of storing data in rows and columns, a knowledge graph is an assembly of concepts and relationships displayed as nodes and edges that form a highly connected graph. An important benefit for the following discussion is that each concept is uniquely identifiable, which allows you to tell that one concept is the same as another. Facts (data) and models (metadata) are represented in the same queryable format, which enables organizations to manage and leverage their knowledge and information across disparate data domains and effectively offers compelling benefits like
- Knowledge discovery, enabling employees to find relevant information faster while improving access and reuse of information.
- Data integration and interoperability, allowing organizations to connect and integrate diverse data sources seamlessly.
- Significantly enhancing an organization’s decision-making processes by providing contextual information and semantic relationships.
- Enhancing search and recommendation systems.
- Enabling efficient knowledge sharing and collaboration across domains and disciplines.
Data Governance (DG)
Organizations that gain a competitive edge are those that connect ALL their internal and external data together. By integrating disparate data sources and overcoming tribal vocabulary, businesses gain a far more comprehensive view of their operations, customers, and markets, enabling them to uncover valuable insights that would otherwise remain hidden. At the same time, the behavior around the definition, production, and usage must be formalized to manage risk and improve the quality and usability of the selected data.
Linking Knowledge Graphs and Data Governance
Enterprises may consider semantic and Enterprise Knowledge Graph technology for their Data Governance efforts since it is essential for them to fully connect the entirety of their existing data for its use and reuse. This approach raises and ensures data quality, enforcing an end-to-end view on data and data lineage while obeying all regulatory requirements, such as data protection, privacy, security.
The diversity of enterprise perspectives on data, namely
- Business
- Regulatory
- Technical
needs to be linked to abstract information models, vocabulary management, metadata management, reference data, business glossary and physical data stores.
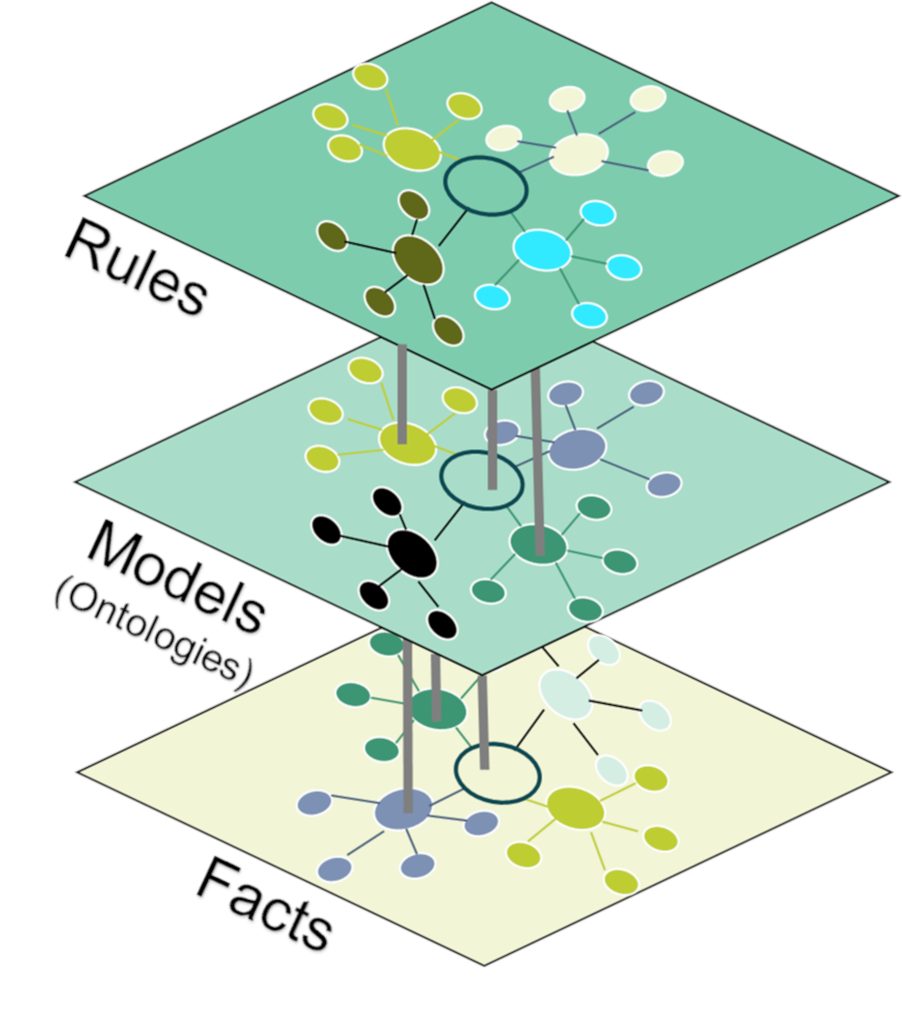
Such an integration contributes to a project’s (or program’s) success by enabling accurate and efficient information extraction and processing. Moreover, it becomes clear that the benefits mentioned before generate value for customers while supporting the application of implicit data governance and therefore keeping the burden away from data users (computational governance), giving them the freedom to focus on their main business goals.
Data Governance in Pharma
Organizations can face significant problems when interpreting standards and terminologies inconsistently across various regions and jurisdictions. The lack of semantic alignment among regulatory bodies means that different contexts may have different meanings and identifiers, even though regulatory compliance requires consistent identities. This can lead to large integration and interoperability costs. Rather than focusing on developing new medications, organizations spend lots of time on data wrangling, e.g., the need to map substance or product data across the organization throughout the product lifecycle.
Consider a scenario where a user asks questions about medicinal product information gathered from internal and external sources. Prerequisites to receive consistent answers across the pharmaceutical data value chain are:
Interoperability among global regulatory and healthcare communities for an accurate analysis and effective communication across jurisdictions. Reporting of adverse events must use a standardized set of product definitions, resulting in higher quality data for signal management. This will also facilitate faster communication, decision-making, and regulatory actions. Standardizing regulatory data management is critical for regulatory compliance. This promotes efficiency, consistency, and accuracy in the regulatory process. This valuable regulatory information can be shared and reused across different procedures and regulatory bodies. Clinical trial data must be accessible to stakeholders through established and uniform standards. This will enhance the evaluation and scientific assessment of medications, as well as promote transparency and effective communication. Manufacturing site inspections rely on easily accessible information, making inspections more efficient, especially in cases of urgent defects. Furthermore, the implementation of consistent data standards can aid in the quick identification of counterfeit
medicines.
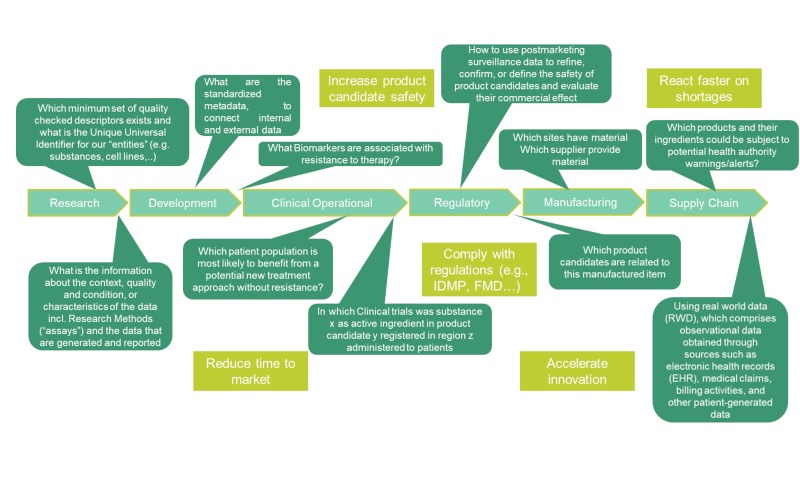
Benefits for Data Governance by combining Knowledge Graphs with LLMs
As described above, a knowledge graph enables description of an area of interest from the real world precisely and unambiguously, resulting in a harmonized and standardized way of representing knowledge and leading to a greater level of interoperability. Nowadays, there are several cross-industry approaches for developing knowledge graphs, driven by ontologies modelling various areas within the pharmaceutical space. An ontology is the standard way of representing domain knowledge as a data model and rule set that data adheres to in a knowledge graph. This ontology driven knowledge graph can act as a crystallization point within a company, allowing different departments to exchange information seamlessly. This approach leads to a common language across the entire pharmaceutical value chain, as visualized in Fig 2. Many case studies have demonstrated this value across a variety of use cases such as identification of medicinal products as shown in Fig 3.
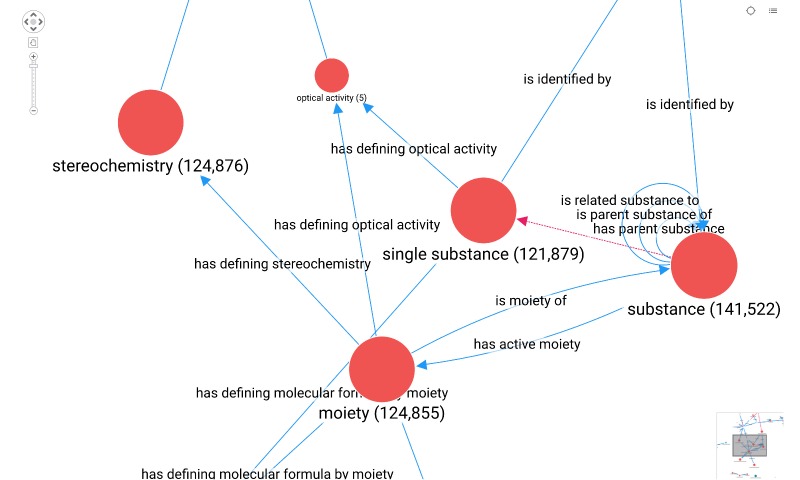
Accessing this knowledge in a self-service way normally involves being able to write queries and a solid understanding of the ontology, which can represent a significant hurdle for data citizens.
Large Language Models (LLMs) can be the drop of oil in the Knowledge Graph machinery, reducing this friction. They can serve as translators, but it’s important to keep in mind that they have their limitations. One of their major drawbacks is producing false or inaccurate results when used alone.
When combined with a Knowledge Graph, it can help data consumers by translating their natural language questions into queries. The resulting output is then translated back into natural language as an answer.
Hence, those two technologies in collaboration take the best of two worlds: making knowledge easily accessible to a broad user group, while retaining the accuracy of a knowledge graph and the ability to explain the results.
Conclusion
All this leads to higher precision and quality, more trust through explainable results, and reduced risk due to explicit data provenance. These all are crucial characteristics that build good data governance in an enterprise. Ultimately, good data governance is essential for maintaining the trust of stakeholders and for achieving organizational goals and objectives.
Disclaimer: The opinions expressed in this article belong solely to the authors and may not necessarily represent the views of any company they are/were associated with.
About the Authors
Dr. Jörg Werner has 30 years’ experience working in the pharmaceutical industry. Since 2016, he has been involved in planning and implementation of various IDMP projects. His focus is on maximizing the value of data through an effective data strategy, management, and governance and establishing a shared, integrated enterprise data environment reflecting business requirements.
Ankit Geete has ten years of experience working in the pharmaceutical industry in different roles. He has a unique skill set combining pharmaceutical science, data, and technology. His aim is to generate business value through data and innovation. His background enables him to demonstrate leadership to innovate and contribute towards enterprise data and digitalization projects/programs.
Michael Lamprecht has been working for a variety of top-tier pharmaceutical companies and has held roles in many areas of the value chain. While driving several data analytics and data governance projects in the last years, he has developed a passion for Knowledge Graphs. As a biochemist who started his professional career in R&D, he is firmly rooted at the origin of value creation in pharma, which helps him translate business problems into data driven solutions.